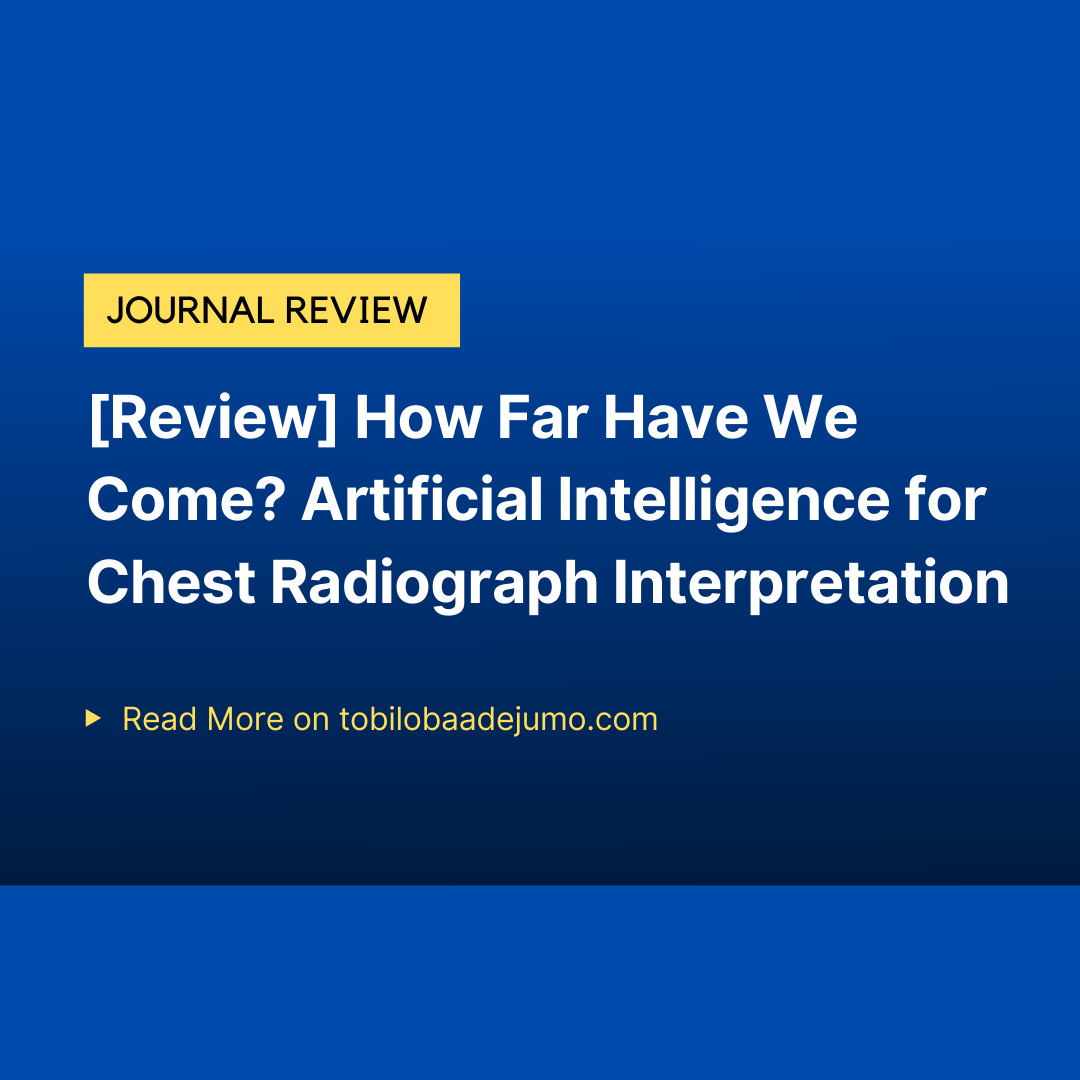
[Review] How Far Have We Come? Artificial Intelligence for Chest Radiograph Interpretation
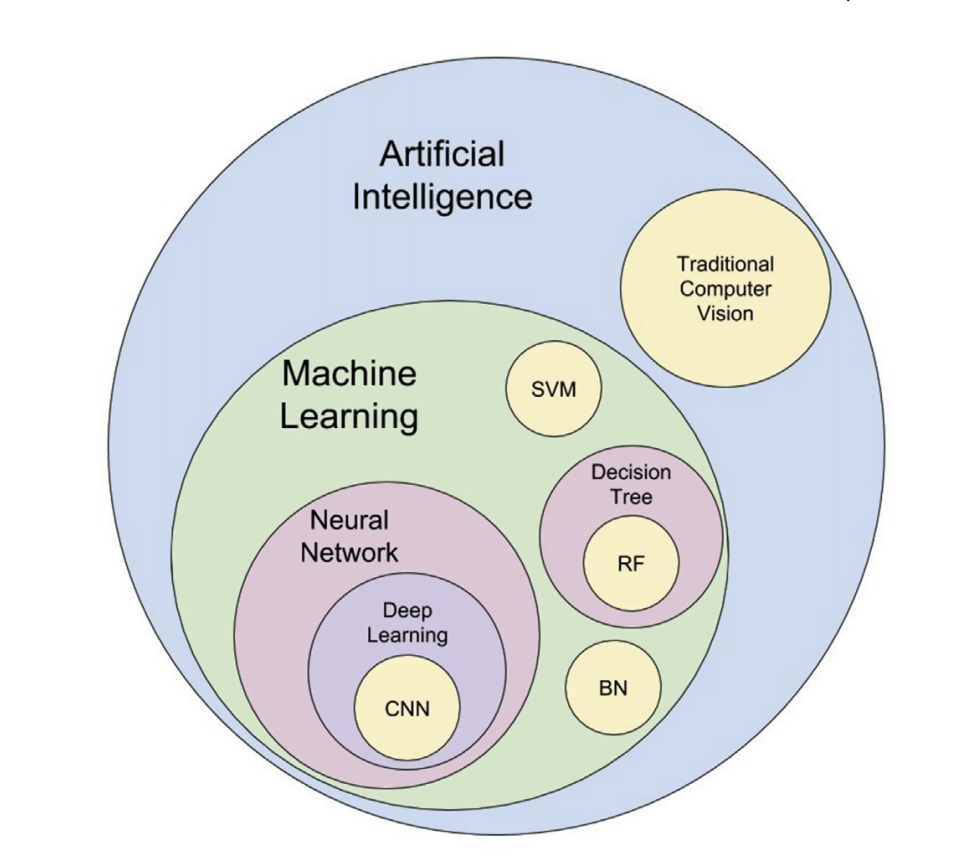
This is my attempt to write and explain using my own words the paper published in Elsevier in 2019. This paper discusses the limitations and applications of artificial intelligence with respect to clinical interpretation of chest radiograph. Here is a link to the paper:
https://pubmed.ncbi.nlm.nih.gov/30704666/
Recognition and appreciation
Special appreciations to the authors of the paper: K. Kallianos, J. Mongan, S. Antani, T. Henry, A. Taylor, J. Abuya, M. Kohli
Disclaimer
If you do not know why I am doing this, you can read my previous article that meticulously tries to explain the purpose of this article.

Table of Contents
1. Recognition and appreciation
2. Introduction
3. Applications of Machine Learning in Chest Radiography
4. Limitations of Artificial Intelligence in a Clinical Setting
Introduction
In the year 1956, the buzzword “artificial intelligence” was coined by a six-man team that proposed a workshop to discuss the past, present and future of artificial intelligence. Since then, artificial intelligence has grown from just being a buzzword to being used in our everyday lives with applications in medicine, engineering, transportation &c.
DeepAI defines machine learning, a subset of artificial intelligence, as a field of computer science that aims to teach computers how to learn and act without being explicitly programmed. In order to be able to this, previous instances of being able to learn and act must be available to the computer so that a model can be built through experience and used in new instances.
In medicine especially, machine learning requires copious amount of data in order to be able to learn and act by predicting unseen data. Over the years, there has been advances such as the introduction of convolutional neural networks (CNN) which is a type of deep learning and publicly available image dataset (contain million of images) which significantly led to the introduction of GPUs. GPUs have more core than a regular CPU. This makes training four to five times faster than a regular CPU. CNN are commonly used for image classification and recognition tasks because of its high accuracy as it is made up of multiple feedforward layers.
Applications of Machine Learning in Chest Radiography
Applications of machine learning in the orientation of images in chest radiographs has been met with a significant level of progress. However, segmentation or separation of lung parenchyma in order to diagnose the parenchyma diseases has been a challenge because the edges of the ribs and clavicles makes it difficult for segmentation to occur. Also, the presence of abnormalities that may be subtle and localised makes it a lot more difficult to detect and juxtapose with previous datasets. These abnormalities also makes it difficult in the screening of tuberculosis because of the variety of imaging manifestations in different anatomical locations
There has been the development of several algorithms that not only assists in the identification of pathogens but also with the localisation of abnormalities. These algorithms have been met with significant progress. The famous work of Li et al suggested that using more annotated medical images during training phase, there is a greater chance of better localisation of images. Find out more about the various types of annotation techniques here
Limitations of Artificial Intelligence in a Clinical Setting
- Limit in generalisation: Algorithms perform best on data that are very similar to training data. ImageNet is mostly used for classification tasks in a clinical setting using transfer learning. Given that not all features in ImageNet can be transferred in clinical settings and the lack of annotated images in ImageNet that can be useful in some medical classification tasks, there is a limit in generalisation. Also because of the number of available medical analysis dataset, it is possible that there will be a data shared (same patient or same study) between the test and train dataset.
- Limit in explainability or explicability: For classification tasks, it is impossible to explain the interaction of thousand pixels to understand why a particular classification binary was chosen over the other. This also applies to segmentation and detection tasks as the boundary box during testing phase doesn’t explain the process of selection.
Further readings
Tobiloba Adejumo Newsletter
Join the newsletter to receive the latest updates in your inbox.